Artificial intelligence — friend or foe of business?
Cyber threats and security systems are evolving rapidly, and artificial intelligence (AI) is playing an important role. Its impact is particularly noticeable in the financial sector, where billions of dollars in potential losses from cyberattacks are being counted. Equally, dramatic are the threats to physical security posed by AI decisions.
Artificial intelligence has become a dual-use technology in the world of cybersecurity — what protects some becomes a weapon in the hands of others. For example, in 2023, Visa’s artificial intelligence system prevented 80 million fraudulent transactions worth $40 billion. At the same time, AI tools like ProKYC, which can create fake identities to bypass verification systems, have emerged in the shadow market.
As a service provider and developer of AI solutions in cybersecurity, we witness this technological duel between defenders and attackers on a daily basis. Our experience shows: the one who uses AI technologies smarter wins.
In our previous article on this topic, we detailed the risks in the development and application of artificial intelligence, including the problems of reliability, confidentiality and integrity of data, as well as the correctness of decisions made by the machine. We’ve also looked in detail at methods for securing AI systems from the very beginning of their development.
Now it’s time to look at security and AI issues from the perspectives of not only AI developers, but also users of AI-based services and solutions. Let’s find out how AI is becoming a shield for modern business.
In this article, we will look at specific examples of real-life cyber incidents involving AI and show how businesses can minimize their risks by relying on smart approaches to information security using AI.
The dual nature of technology
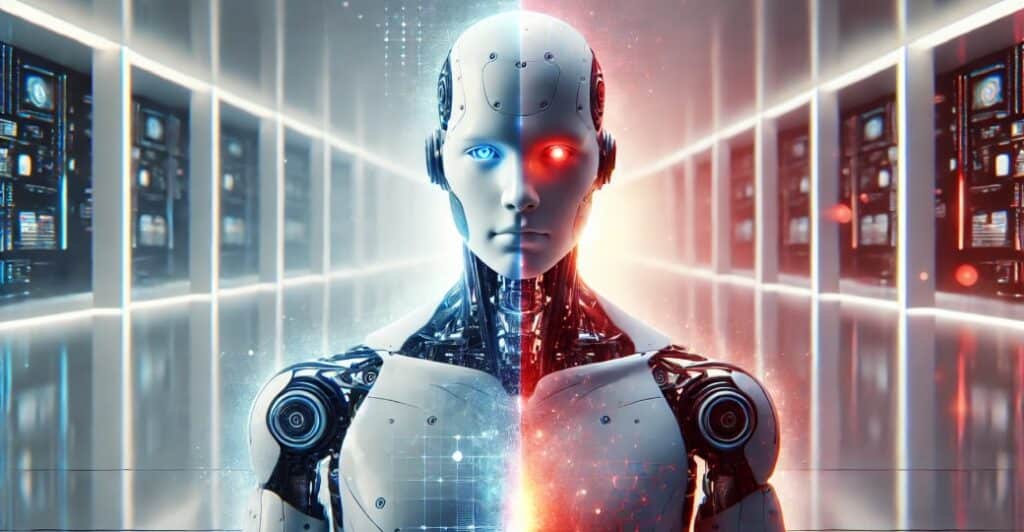
Modern technology has long had a dual nature: it can both enhance defenses and cause new risks and incidents. This was evident long before the explosion of AI in recent years. For example, encryption protects sensitive data in transmission and storage, reducing the risk of leaks, but attackers also use strong encryption and anonymization to conceal criminal activity, making it more difficult to investigate incidents and cybercrime.
As another example, through its distributed nature, blockchain increases data security, integrity and transaction transparency, but reduces the security of some processes due to the irreversibility of erroneous transactions, risks of loss of access keys, smart contract vulnerabilities and other inherent blockchain specifics.
Artificial intelligence (AI) is a prime example of this phenomenon. In the hands of experts, AI can improve the security of companies by predicting potential attacks, analyzing large amounts of data, and identifying anomalies. If misused, AI can be used to automate hacker attacks and other unwanted activities. Again, however, proper implementation of defenses, including those using AI, can effectively counter such attempts. Let us consider the main facets of the dual nature of AI in the modern view.
AI as a business advocate
According to Gartner’s report “Hype Cycle for Artificial Intelligence 2024,” AI enhances business security through three key mechanisms:
- Intelligent threat detection. AI analyzes large volumes of data in real time. This allows it to identify anomalies and potential threats much faster and more accurately than traditional methods.
- Automate response. Modern AI solutions are capable of automatically taking protective measures when threats are detected. This significantly reduces the burden on security teams and speeds up incident response.
- Behavioral analysis. AI systems track and analyze user actions. This helps in detecting suspicious activity and potential insider threats in a timely manner.
If you look at the evolution of modern cybersecurity solutions, the application of AI can be seen in so many classes of modern security systems – from analyzing files and traffic for attacks and malware to managing cloud environments and responding to malicious user actions.
AI as a source of threats
At the same time, Gartner points to serious risks associated with the use of AI:
- The evolution of cyber threats. Attackers are using AI to create more sophisticated attacks, including generating compelling phishing messages and automating hacking processes.
- The false alarm problem. Poorly designed or misconfigured AI systems can generate too many false alarms. This leads to poor utilization of security resources.
- Ethical and legal challenges. The use of AI raises serious issues of data privacy, possible bias in algorithms, and allocation of responsibility for automatically made decisions.
For technology to work for good, it is important to implement it with cybersecurity in mind and regularly assess its potential risks. Businesses should invest not only in AI development, but also in training employees, developing effective incident response strategies, and implementing layered defense solutions. Let’s discuss this in more depth.
Real-life AI cyber incidents: analysis and business impact
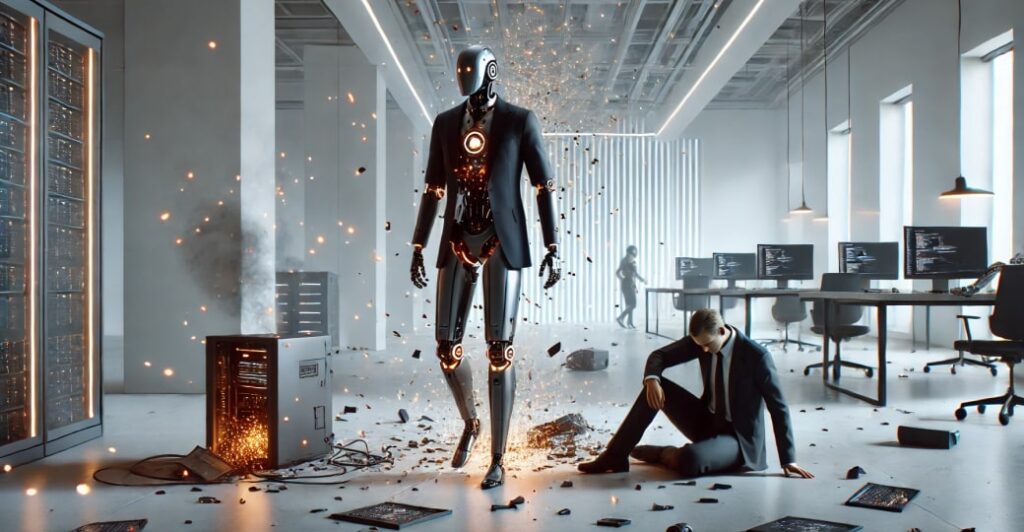
Let’s take a closer look at specific cases that highlight the importance of AI security and its impact on business.
AI is a killer (2018)
The history of artificial intelligence development contains examples that demonstrate not only the vulnerability of advanced technologies, but also the physical security risks that are caused by these technologies. Particularly alarming are situations where failures in AI systems have serious consequences for human life and safety. In 2018, there was a tragic incident involving an unmanned Uber car: the AI system failed to correctly identify a pedestrian, leading to a fatal collision.
Business Risks: If your business is related to autonomous transportation, IoT, IIoT, operational technology, and other applications of computer technology in the physical world, it’s only a matter of time before AI technologies are incorporated into your processes or products. Accordingly, don’t passively wait for AI-related cyber-physical incidents, but prepare to manage these risks now.
Voice Deepfake Fraud (2019)
In March 2019, the CEO of a UK division of an energy company received a call from an individual imitating his boss’s voice using deepfake technology. The attacker convinced him to transfer £200,000 (about USD 243,000) to an alleged Hungarian supplier.
This case was one of the first high-profile incidents to demonstrate how AI can be used to create fake votes for financial fraud. Further developments in deepfake technology have shown that in addition to voice spoofing, fraudsters are also effectively using fake videos, including real-time video, to deceive their victims.
Business risk: the threats of deepfakes are forcing a rethinking of authentication procedures that previously relied on voice or video confirmations. A major problem may lie in the fact that not all such procedures are formally described. Companies often have unwritten procedures and informal practices related to various personal requests or confirmations.
Clearview AI facial recognition hack (2020)
Clearview AI, a developer of facial recognition technology for law enforcement and private companies, experienced an attack in which hackers stole customer and employee data. Given that biometric data is unique and cannot be changed like a password or phone number, the leak has sparked public concern and discussions internationally.
Business implications: the incident has led to legal disputes and regulatory inspections. The loss of biometric data can make it impossible to use several services, as such data cannot be re-generated. For businesses, this signals the need for strict security measures and additional levels of biometric data encryption.
Manipulation of YouTube’s recommendation system (2020)
In 2020, researchers discovered that YouTube’s recommendation system had been manipulated: attackers used bots to artificially increase the number of views and interactions with content. This led to the spread of misinformation and malicious content, harming users and drawing criticism of the platform. Such manipulations demonstrated the vulnerability of algorithms to metrics and activity spoofing. This has increased distrust in the recommendation system and information platforms in general.
Business risks: rating manipulation and misinformation can cause significant damage to a brand’s reputation. For example, promoting false information about a company can provoke a drop in stock or distrust from customers.
ChatGPT conversation headers leak (2023)
In March 2023, a ChatGPT incident occurred: users noticed that other people’s conversation headers were displayed when they opened the chat room. This was due to a glitch in the open source library used by the application. OpenAI quickly fixed the issue and implemented measures to prevent the error from recurring.
Business impact: this incident underscored the importance of data security in AI-based services. Leaks of this nature can undermine user trust, lead to legal repercussions and financial losses. According to IBM’s Report for 2024, the average loss to companies from a data breach was 4.88 million dollars. While OpenAI’s exact financial losses were not disclosed, the incident likely resulted in additional costs to strengthen security measures, settle potential claims, and repair reputations. Such costs are often included in the amount of damage from an incident.
ChatGPT conversation histories leaked for macOS (2024)
In July 2024, users discovered that the ChatGPT app for macOS was storing conversation histories on local devices in unencrypted form. This meant that anyone with physical access to the device could view the contents of conversations, including sensitive information such as corporate data or private conversations.
OpenAI responded quickly and released an update, implementing local data encryption to address the vulnerability. However, the incident was a reminder of the importance of securing AI applications at the user device level.
Conclusion: for businesses, this case highlights the need for regular audits and verification of AI software security settings. Implementing local drive monitoring tools and centralized application security management can prevent such incidents.
An AI-related security incident can be not just a technology problem, but also a reputational blow to companies. Leaks of sensitive information can cause irreparable damage to the trust of customers and partners. Businesses that rely on AI risk facing consequences such as drop in sales, regulatory proceedings and even the departure of key partners.
Data confidentiality is under special scrutiny, as any leakage of information can cause a serious scandal. In addition, a breach of data reliability and integrity complicates decision-making: business processes start to be based on distorted or false information.
To reduce such risks, businesses should reconsider all their processes from the point of view of new threats and implement comprehensive security measures, including protection against external and insider threats, and AI’s erroneous decisions.
Popular ways to improve business security with AI
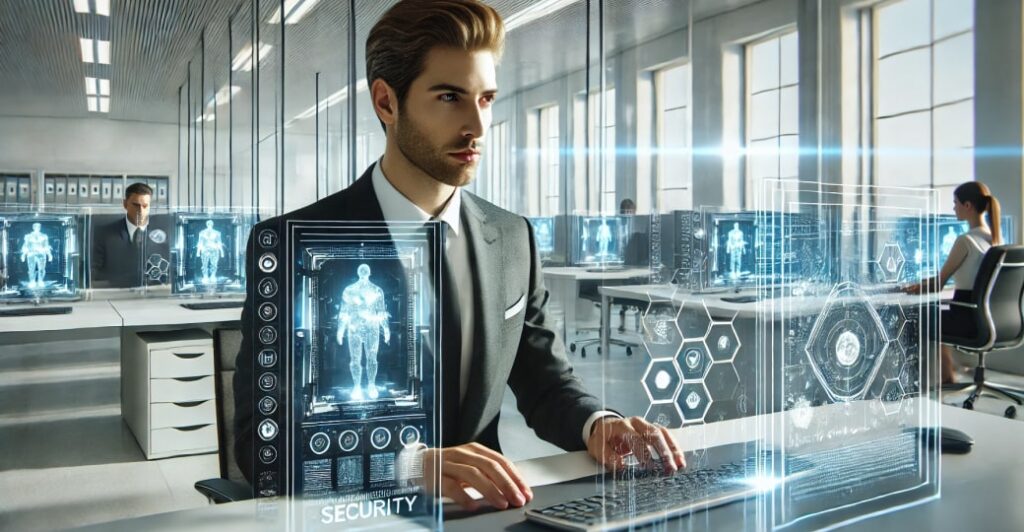
As we mentioned above, many technologies, if not almost all, can both improve business reliability and security and reduce it. In the previous and current articles, we have paid enough attention to the security risks of AI. So now let’s move on to the opportunities to improve business reliability and security that AI presents to us.
Let’s take a look at the most effective, actively used modern methods of improving IT security with the help of AI and give real examples of their successful application.
1. Malware detection and blocking: smart defense systems
One of the main challenges of information security is detecting and preventing malware (software) infiltration. Modern security systems use a combination of signature analysis, behavioral analysis, and AI-based predictive analytics, often integrated with cloud-based security systems.
AI has greatly improved the process of detecting such threats. Instead of pattern-based analysis, machine learning (ML) algorithms identify abnormal system behavior that could indicate malicious activity.
Example: Palo Alto Networks has developed a platform capable of identifying new types of attacks by analyzing file behavior and network traffic. In one case study, this system prevented an attack using a virus that masqueraded as a routine program update.
Other companies, such as Darktrace, are using AI to create “digital immunity,” a model that recognizes anomalies based on how a “healthy” system should function. This allows threats to be blocked before they are even actively manifested.
2. Optimizing Identity and Access Management
Complex systems with multiple users are often vulnerable due to poor access controls and human error. Forgotten accounts, misconfigured access rights, and employees using the same passwords all increase the risk of data leakage and hacking.
AI helps create a layered access control system by analyzing user sessions and identifying suspicious behavior.
Case: Azure Active Directory from Microsoft applies AI to monitor login attempts. If the system detects suspicious activity, such as an attempt to log in from an unfamiliar location or at an unusual time, it blocks access and notifies an administrator. This prevents hundreds of millions of attacks around the world every day.
3. Automating incident response processes
In the face of cyberattacks, every second can make a difference. Rapid incident response can minimize the impact and prevent the threat from spreading to other systems. However, without automation, many companies lose valuable time due to manual checks and approvals.
AI systems are able to automatically recognize an incident, isolate the infected network segment, and activate response scenarios without requiring human intervention.
Example: IBM uses AI-based solutions that can reduce incident handling time by 75%. In a case study, the company prevented a massive attack on its data centers by automatically blocking suspicious traffic and alerting the security service about the need for detailed analysis.
4. Integrating generative AI into administrative interfaces: enhanced transparency and analytics
Generative AI in administrative security dashboards allows you to analyze vast amounts of data and present it in a way that is easy to understand for administrators. This helps to detect patterns that might have gone undetected with a traditional approach.
For example, generative models such as ChatGPT can offer the administrator scenarios to optimize security policy based on current data and past incidents. Instead of manually searching for problems, the system independently formulates suggestions and steps to improve security.
Case: Splunk has implemented integration with generative systems that provide analytic reports and recommendations to quickly remediate vulnerabilities.
5. Vulnerability Patch Prioritization: Smart Solutions for Rapid Patching
In large companies, the number of vulnerabilities in systems can number in the thousands, and not every vulnerability can be fixed instantly. Incorrect prioritization can lead to a truly critical vulnerability being overlooked.
AI helps identify which vulnerabilities pose the greatest risk to the business and suggests how to address them. Prioritization systems use contextual data – for example, the likelihood of a vulnerability being exploited and its impact on key company functions.
Success story: Qualys implemented an AI-based predictive vulnerability analysis system. As a result, the time to assess and remediate critical vulnerabilities was reduced by 40%. This reduced the likelihood of successful attacks on the company’s infrastructure.
6. Personalized security training
Traditional information security training is often ineffective because it provides employees with general information without taking into account their behaviors and actions. AI makes it possible to create customized training programs by analyzing each employee’s computing habits and knowledge level.
Examples: platforms Immersive Labs and CybSafe use algorithms that generate training scenarios based on how an employee interacts with corporate systems. If the AI notices that a user is prone to opening suspicious emails or using insecure sites, they are offered specific training on phishing and threat recognition.
Benefit: a personalized approach reduces the likelihood of employee error and transforms them from a weak link to an active line of defense.
7. Other popular ways and successful cases
AI helps identify employee actions that could threaten security, whether it’s unauthorized access to data, accidental or intentional attempts to bypass security systems. For example, the Forcepoint system applies behavioral analytics to track unusual activity in the corporate environment.
To counter phishing threats, companies are actively deploying AI-based solutions. For example, Darktrace has developed an AI system capable of detecting and blocking phishing attacks in real time by analyzing user behavior and network traffic.
AI algorithms capable of analyzing network traffic and detecting malicious activity are used to combat bots. For example, Cloudflare introduced an AI-based tool to protect websites from bots that unauthorizedly extract data to train artificial intelligence models.
The application of AI in information security opens up a wide range of opportunities for businesses to protect data and prevent cyberattacks. Companies that implement modern solutions are able to quickly identify and block threats, improve access control and automate incident response processes. However, it is important to realize that AI technologies are not a panacea. Without a comprehensive approach and constant security monitoring, even the most advanced algorithms may be powerless against cyber threats.
Promising ways to improve information security
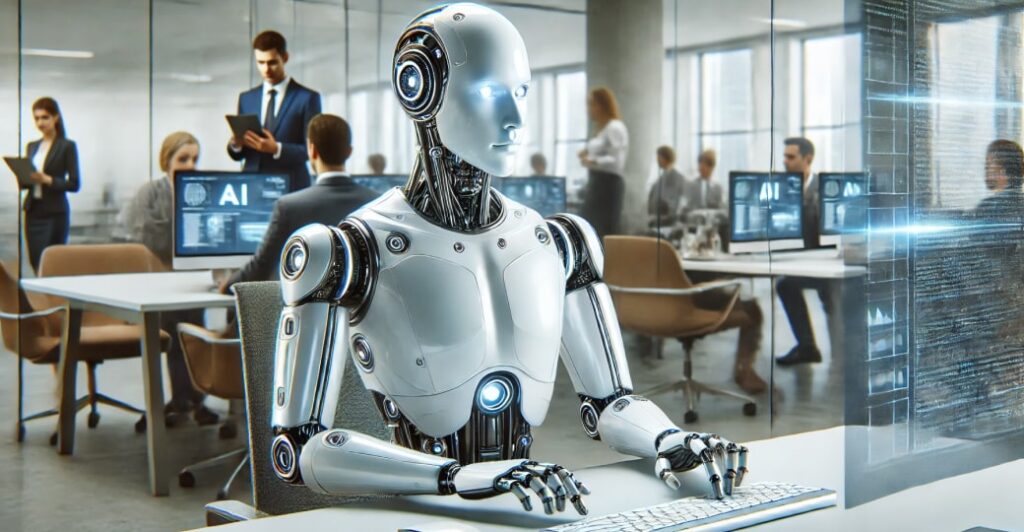
To keep pace, companies must not only utilize current AI technologies, but also introduce innovative approaches. Let’s take a look at promising trends that can strengthen information security and minimize future risks.
Predicting future attacks
One of the main challenges of information security is not just responding to incidents that have already happened, but predicting potential threats. Traditional systems focus on detecting known attack patterns. Modern AI goes further: it creates predictive models that analyze attacker behavior and global trends. This allows vulnerabilities to be identified before they are exploited.
Example: the CrowdStrike platform uses advanced predictive analytics technology. The system collects data on millions of cyberattacks worldwide, applies machine learning algorithms to identify hidden patterns, and creates dynamic behavioral scenarios. This allows it not only to detect known threats, but also to predict new attack vectors. In one of the cases, such a system helped prevent a large-scale phishing attack by detecting its signs even before the mass mailing began, based on the analysis of the attackers’ preparatory actions.
Future applications: the next stage of development will be “digital predictors” that can not only identify general trends, but also warn of threats with pinpoint accuracy to specific systems and attack directions using next-generation neural networks.
Identifying weaknesses in cybersecurity defenses
It’s not uncommon for companies to believe their systems are fully protected until an attack occurs. But AI systems can help identify weaknesses before attackers exploit them. Automated tools run simulations of attacks and assess how systems respond to them to identify “gaps” in defenses.
Example: the AttackIQ solution allows companies to simulate cyberattack scenarios and test their infrastructure for resilience. This helps to see in real time where a system is vulnerable and remediate those issues before real threats emerge. Such analysis is much deeper than vulnerability scanning. This is roughly the kind of modeling that Pentesters and Red Teams do, except they do it sporadically, like once a year or quarter, while AI-based systems can test the security of companies continuously and around the clock.
Self-adaptive security systems
Self-adaptive systems are one of the most promising steps in the development of cybersecurity. Such systems are able to analyze threats and rebuild their defense algorithms in real time, adapting to new types of attacks without human intervention.
Example: Darktrace has developed an AI model that not only blocks threats, but also “learns” from each incident, updating its defense model. Such a system, noticing an anomaly in user or network behavior, can instantly change its access policy and block a potential attack vector.
Future: with the development of such systems, companies will be able to obtain protection that “evolves” with cyber threats and minimizes the factor of outdated security rules.
Development of quantum-resistant encryption methods
With the development of quantum computers, traditional encryption algorithms such as RSA and AES may become vulnerable. AI plays a key role in developing new security methods that will be resilient to the capabilities of quantum computing.
Fact: companies such as IBM and Google are already actively testing quantum-resistant algorithms, using AI to analyze their robustness and resilience to attacks.
In the future, this will lead to encryption that can protect data even with quantum computing power that can crack current algorithms in minutes.
Global cybersecurity collaboration
The complexity of today’s cyber threats requires coordinated efforts from companies, governments and information security solution providers. AI has the potential to help create global platforms for sharing data on cyberattacks and defense techniques to rapidly deploy solutions around the world.
Example: the international Cyber Threat Alliance initiative brings together companies around the world and uses AI to analyze and disseminate information about emerging threats. This makes it possible to prevent attacks before they reach certain regions.
Other promising directions
Some other promising areas of application of AI in cybersecurity:
- Eliminating false positives. Developing AI systems that can reduce false positives and provide only reasonable signals.
- AI to protect IoT devices. With the rise of smart devices, AI is helping prevent attacks on connected sensors, cameras, and appliances.
- Ethical auditing of algorithms. Implementing AI to ensure that security algorithms do not make biased decisions and meet standards of fairness and transparency.
The options for applying AI in cybersecurity are not limited to the examples given. The work of an information security professional involves a lot of routine work that has long been automated. However, there is still a lot of intellectual work that cannot be automated by traditional means, but can be made easier with AI.
A final look at the role of AI in business cybersecurity and recommendations
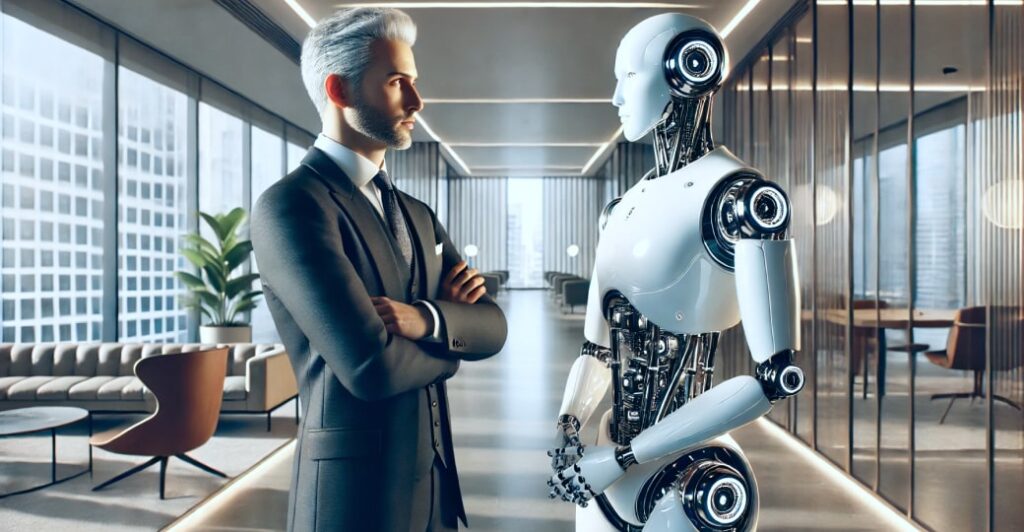
The use of AI in information security holds many promise for companies. AI can automate processes, provide accurate threat detection, and even predict potential attacks. However, complete reliance on technology without human oversight can lead to unintended consequences, such as errors in AI models or poor decision-making when false positives are detected.
With each new step in the development of AI technology, the need to find a balance between AI’s capabilities and its potential risks increases. So how can businesses find the “golden mean” and use AI effectively without compromising security?
Balancing opportunities and risks
To effectively utilize AI, it is important to follow three key principles:
- Integrating AI with human controls. The effectiveness of AI systems is enhanced with regular audits and assessments, which can be conducted by either internal specialists or external experts, depending on the organization’s capabilities and needs.
- Invest in employee training. People remain the most important link in cybersecurity. Training on AI systems and incident response scenarios is an important step toward reducing human error.
- Developing an AI implementation strategy. Before integrating AI into security, it is important to conduct a comprehensive risk analysis and develop a plan that addresses the technical and legal aspects of data protection.
Recommendations for small and medium-sized businesses
Companies of different sizes have their own peculiarities when implementing AI. For smaller organizations, there are specialized solutions that enable them to leverage the benefits of AI in a way that is tailored to their specific needs. Today’s cloud solutions make advanced technology accessible even to smaller companies, allowing you to protect your business without a large upfront investment.
Our recommendations:
Use cloud-based information security solutions with AI features. For small businesses, this is the optimal choice for several reasons:
- No need for in-house infrastructure
- Pay on a subscription model instead of large one-time investments
- Automatic updates and support from the provider
- Scalability as your business grows
Automate basic security processes. SMBs often can’t afford large IT staffs, so AI-powered solutions can help:
- Automatically track suspicious activity
- Block common threats without human intervention
- Conduct regular security audits
- Generate reports on the state of protection
Implement affordable auditing tools. For smaller companies, it’s important to balance efficiency and cost:
- Use built-in validation tools in cloud services
- Use automated vulnerability scanners
- Perform basic security audits on a regular basis
- Order external security audits from vendors with low-budget plans
Recommendations for corporations
The larger the corporation and the more complex its IT infrastructure, the higher its risk of data breaches and attacks on critical systems. In such an environment, AI becomes a necessity for large-scale monitoring and automation of security processes.
Our recommendations:
Implement AI-based predictive threat intelligence systems. For large businesses, this is critical for the following reasons:
- The ability to analyze massive amounts of data in real time
- Early detection of potential attacks before they occur
- Automatic mapping of threats to business risks
- Prioritize protective measures based on data analytics
Invest in quantum-resistant encryption. For corporations, this is a strategically important area. Though it may not seem to make practical sense right now, post-quantum cryptography can be considered the next level of your security. Benefits:
- Protecting sensitive data from future quantum attacks
- Compliance with forward-looking regulatory requirements
- Ensuring long-term security of corporate secrets
- Prepare your infrastructure for the new technological era
Leverage self-adaptive AI-based security systems. The scale and complexity of today’s enterprise infrastructure requires the following optimization of security processes:
- Automatically adapting to new threats in real time
- Continuous learning based on incident analysis
- Proactive defense against unknown types of attacks
- Automatically adjust security policies
Create AI-enabled internal response centers (SOCs) or use external SOC services. Large organizations need this for:
- Centralized monitoring of the entire infrastructure
- Rapid incident response 24/7
- Automate threat investigation and remediation
- Continuous improvement of security processes
Every company is unique, so the implementation of AI in its security processes should take into account the specifics of the industry, technical infrastructure and business goals. When planning implementation, it’s important to consider not only current needs, but also the organization’s growth prospects so that the selected solutions can scale with business growth.
Implementing AI in enterprise security may seem like a daunting task, but with the right approach, it will give the company a significant advantage over competitors and protect key data. It is recommended to start by assessing the current level of security, identifying critical assets and gradually implementing AI systems, starting with the highest priority areas.
To successfully implement an AI-based security strategy, it is worth turning to specialized information security companies. A professional audit of your existing infrastructure and expert support during implementation will help you avoid common mistakes and maximize the effectiveness of your security investment.
Leave request on our website and get free professional consultation, AI implementation strategy development, and proper secure solution integration.
_________________________
Subscribe to us at x.com so you don’t miss our news and blog articles.